![]() |
![]() |
![]() |
Nearest Neighbor Embeddings Classification with QdrantΒΆ
FiftyOne provides powerful workflows centered around embeddings, including pre-annotation, finding annotation mistakes, finding hard samples, and visual similarity searches. However, performing nearest neighbor searches on large datasets requires the right infrastructure.
Vector search engines have been developed for the purpose of efficiently storing, searching, and managing embedding vectors. Qdrant is a vector database designed to perform an approximate nearest neighbor search (ANN) on dense neural embeddings, which is a key part of any production-ready system that is expected to scale to large amounts of data. And best of all, itβs open-source!
In this tutorial, weβll load the MNIST dataset into FiftyOne and then use Qdrant to perform ANN-based classification, where the data points will be classified by selecting the most common ground truth label among the K nearest points from our training dataset. In other words, for each test example, weβll select the K nearest neighbors in embedding space and assign the best label by voting. Weβll then evaluate the results of this classification strategy using FiftyOne.
So, whatβs the takeaway?
FiftyOne and Qdrant can be used together to easily perform an approximate nearest neighbors search on the embeddings of your datasets and kickstart pre-annotation workflows.
SetupΒΆ
If you havenβt already, install FiftyOne:
[ ]:
!pip install fiftyone
Weβll also need the Qdrant Python client:
[ ]:
!pip install qdrant_client
In this example, we will also be making use of torchvision models from the FiftyOne Model Zoo.
[ ]:
!pip install torchvision
Qdrant installationΒΆ
If you want to start using the semantic search with Qdrant, you need to run an instance of it, as this tool works in a client-server manner. The easiest way to do this is to use an official Docker image and start Qdrant with just a single command:
[ ]:
!docker run -p "6333:6333" -p "6334:6334" -d qdrant/qdrant
After running the command weβll have the Qdrant server running, with HTTP API exposed at port 6333 and gRPC interface at 6334.
Loading the datasetΒΆ
There are several steps we need to take to get things running smoothly. First of all, we need to load the MNIST dataset and extract the train examples from it, as weβre going to use them in our search operations. To make everything even faster, weβre not going to use all the examples, but just 2500 samples. We can use the FiftyOne Dataset Zoo to load the subset of MNIST we want in just one line of code.
[1]:
import fiftyone as fo
import fiftyone.zoo as foz
import fiftyone.brain as fob
[3]:
# Load the data
dataset = foz.load_zoo_dataset("mnist", max_samples=2500)
train_view = dataset.match_tags(tags=["train"])
Split 'train' already downloaded
Split 'test' already downloaded
Loading 'mnist' split 'train'
100% |βββββββββββββββ| 2500/2500 [4.1s elapsed, 0s remaining, 685.3 samples/s]
Loading 'mnist' split 'test'
100% |βββββββββββββββ| 2500/2500 [4.0s elapsed, 0s remaining, 644.8 samples/s]
Dataset 'mnist-2500' created
Letβs start by taking a look at the dataset in the FiftyOne App.
[34]:
session = fo.launch_app(train_view)
[35]:
session.freeze() # screenshot the App
Generating embeddings and loading into QdrantΒΆ
The next step is to generate embeddings on the samples in the dataset. This can always be done outside of FiftyOne, with your own custom models. However, FiftyOne also provides various different models in the FiftyOne Model Zoo that can be used right out of the box to generate embeddings.
In this example, we use MobileNetv2 trained on ImageNet to compute an embedding for each image.
[4]:
# Compute embeddings
model = foz.load_zoo_model("mobilenet-v2-imagenet-torch")
train_embeddings = train_view.compute_embeddings(model)
100% |βββββββββββββββ| 2500/2500 [3.7m elapsed, 0s remaining, 7.7 samples/s]
As of FiftyOne version 0.20.0, FiftyOne natively integrates with Qdrant! This means that uploading your embeddings is now as simple as computing similarity on your dataset with the qdrant backend.
[ ]:
# Compute similarity with Qdrant backend
fob.compute_similarity(train_view, embeddings=train_embeddings, brain_key="qdrant_example", backend="qdrant")
Alternatively, if you donβt want to precompute your embeddings, you can pass in a model parameter to compute_similarity and the embeddings will be computed for you and stored in the given field name upon completion.
[ ]:
# Compute similarity with Qdrant backend without precomputed embeddings
#fob.compute_similarity(train_view, model = model, embeddings=train_embeddings_new, brain_key="qdrant_example", backend="qdrant")
Nearest neighbor classificationΒΆ
Now to perform inference on the dataset. We can create the embeddings for our test dataset, but just ignore the ground truth and try to find it out using ANN, then compare if both match. Letβs take one step at a time and start with creating the embeddings.
[9]:
# Assign the labels to test embeddings by selecting the most common label
# among the neighbours of each sample
test_view = dataset.match_tags(tags=["test"])
test_embeddings = test_view.compute_embeddings(model)
100% |βββββββββββββββ| 2500/2500 [3.9m elapsed, 0s remaining, 12.1 samples/s]
Time for some magic. Letβs simply iterate through the test datasetβs samples and their corresponding embeddings, and use sort_by_similarity to find the 15 closest embeddings from the training set. Weβll also need to calculate the class counts to determine the most common label. This will be stored as an "ann_prediction"
on each test sample in FiftyOne.
The function below takes an embedding vector as input, uses FiftyOneβs sort_by_similarity functionality to find the nearest neighbors to the test embedding, generates a class prediction, and returns a FiftyOne Classification object that we can store in our FiftyOne dataset.
[13]:
def generate_fiftyone_classification(embedding, brain_key, field="ground_truth"):
search_results = dataset.sort_by_similarity(embedding, k=15, brain_key=brain_key)
# Count the occurrences of each class and select the most common label
# with the confidence estimated as the number of occurrences of the most
# common label divided by a total number of results.
class_counts = search_results.count_values(field + ".label")
predicted_class = max(class_counts, key=class_counts.get)
occurences_num = class_counts[predicted_class]
confidence = occurences_num / sum(class_counts.values())
prediction = fo.Classification(
label=predicted_class, confidence=confidence
)
return prediction
[10]:
from tqdm import tqdm
predictions = []
# Call Qdrant to find the closest data points
for embedding in tqdm(test_embeddings):
prediction = generate_fiftyone_classification(embedding, brain_key="qdrant_example")
predictions.append(prediction)
test_view.set_values("ann_prediction", predictions)
100%|ββββββββββ| 2500/2500 [01:42<00:00, 24.40it/s]
By the way, we estimated the confidence by calculating the fraction of samples belonging to the most common label. That gives us an intuition of how sure we were while predicting the label for each case and can be used in FiftyOne to easily spot confusing examples.
Evaluation in FiftyOneΒΆ
Itβs high time for some results! Letβs start by visualizing how this classifier has performed. We can easily launch the FiftyOne App to view the ground truth, predictions, and images themselves.
[6]:
session = fo.launch_app(test_view)
[7]:
session.freeze() # screenshot the App
FiftyOne provides a variety of built-in methods for evaluating your model predictions, including regressions, classifications, detections, polygons, instance and semantic segmentations, on both image and video datasets. In two lines of code, we can compute and print an evaluation report of our classifier.
[11]:
# Evaluate the ANN predictions, with respect to the values in ground_truth
results = test_view.evaluate_classifications(
"ann_prediction", gt_field="ground_truth", eval_key="eval_simple"
)
# Display the classification metrics
results.print_report()
precision recall f1-score support
0 - zero 0.87 0.98 0.92 219
1 - one 0.94 0.98 0.96 287
2 - two 0.87 0.72 0.79 276
3 - three 0.81 0.87 0.84 254
4 - four 0.84 0.92 0.88 275
5 - five 0.76 0.77 0.77 221
6 - six 0.94 0.91 0.93 225
7 - seven 0.83 0.81 0.82 257
8 - eight 0.95 0.91 0.93 242
9 - nine 0.94 0.87 0.90 244
accuracy 0.87 2500
macro avg 0.88 0.87 0.87 2500
weighted avg 0.88 0.87 0.87 2500
After performing the evaluation in FiftyOne, we can use the results
object to generate an interactive confusion matrix allowing us to click on cells and automatically update the App to show the corresponding samples.
[37]:
plot = results.plot_confusion_matrix()
plot.show()
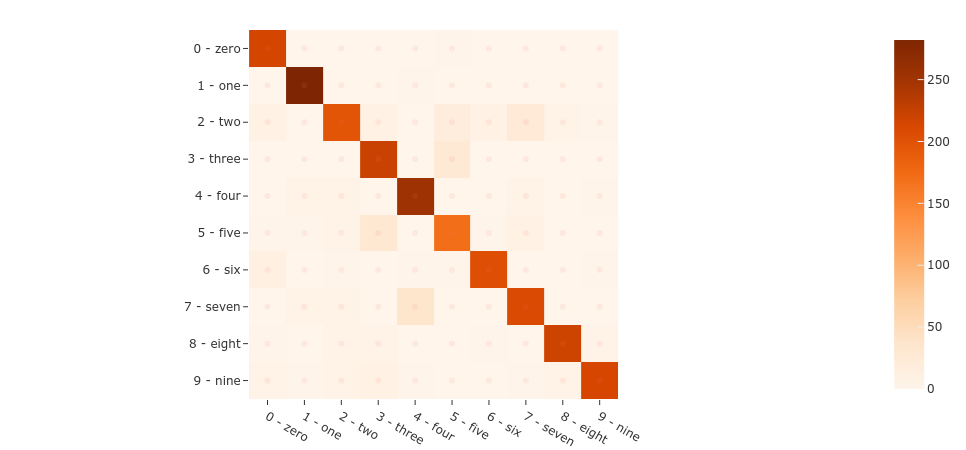
[ ]:
session.plots.attach(plot)
Letβs dig in a bit further. We can use the sophisticated query language of FiftyOne to easily find all predictions that did not match the ground truth, yet were predicted with high confidence. These will generally be the most confusing samples for the dataset and the ones from which we can gather the most insight.
[3]:
from fiftyone import ViewField as F
# Display results in the FiftyOne App, but include only the wrong predictions that were
# predicted with high confidence
false_view = (
test_view
.match(F("eval_simple") == False)
.filter_labels("ann_prediction", F("confidence") > 0.7)
)
[4]:
session = fo.launch_app(false_view)
[5]:
session.freeze() # screenshot the App
These are the most confusing samples for the model and, as you can see, they are fairly irregular compared to other images in the dataset. A next step we could take to improve the performance of the model could be to use FiftyOne to curate additional samples similar to these. From there, those samples can then be annotated through the integrations between FiftyOne and tools like CVAT and Labelbox. Additionally, we could use some more vectors for training or just perform a fine-tuning of the model with similarity learning, for example using the triplet loss. But right now this example of using FiftyOne and Qdrant for vector similarity classification is working pretty well already.
And thatβs it! As simple as that, we created an ANN classification model using FiftyOne with Qdrant as an embeddings backend, so finding the similarity between vectors can stop being a bottleneck as it would in the case of a traditional k-NN.
BDD100k exampleΒΆ
Letβs take everything we learned in the previous example and apply it to a more realisitc use-case. In this section, we take a look at how to use nearest neighbor embedding classification for pre-annotation of the BDD100k road-scene dataset to apply a scene-level label determining the time of day.
This dataset is also available in the FiftyOne Dataset Zoo. If you want to follow along youself, you will need to register at https://bdd-data.berkeley.edu in order to get links to download the data. See the zoo docs for details on loading the dataset.
Weβll be working with a 1,000 image subset of the validation split:
[8]:
import fiftyone as fo
import fiftyone.zoo as foz
# The path to the source files that you manually downloaded
source_dir = "/path/to/dir-with-bdd100k-files"
dataset = foz.load_zoo_dataset(
"bdd100k",
split="validation",
source_dir=source_dir,
max_samples=1000,
)
Split 'validation' already prepared
Loading 'bdd100k' split 'validation'
100% |βββββββββββββββ| 1000/1000 [21.4s elapsed, 0s remaining, 48.2 samples/s]
Dataset 'bdd100k-validation-1000' created
Letβs split this dataset so that 30% of the samples are missing the timeofday
classification. We will then compute this classification using nearest neighbors.
[9]:
import fiftyone.utils.random as four
four.random_split(dataset, {"train": 0.7, "test": 0.3})
train_view = dataset.match_tags("train")
test_view = dataset.match_tags("test")
# Remove labels from test view for this example
test_view.set_field("timeofday", None).save()
Note: This is a larger model than the one used in the previous example. It is recommended this is run on a machine with GPU support or in a Colab notebook.
[10]:
# Load a resnet from the model zoo
model = foz.load_zoo_model("resnet50-imagenet-torch")
# Verify that the model exposes embeddings
print(model.has_embeddings)
# True
# Compute embeddings for each image
train_embeddings = train_view.compute_embeddings(model)
True
100% |βββββββββββββββββ| 700/700 [24.8m elapsed, 0s remaining, 0.5 samples/s]
[21]:
train_embeddings.shape
[21]:
(700, 2048)
From here we can use the functions defined above to load the embeddings into Qdrant and then generate classifications for the dataset. Specifically, we are looking to generate pre-annotations of the timeofday
label.
[11]:
# Compute similarity with Qdrant backend
fob.compute_similarity(train_view, embeddings=train_embeddings, brain_key="bdd100k_qdrant_example", backend="qdrant")
[16]:
test_embeddings = test_view.compute_embeddings(model)
100% |βββββββββββββββββ| 300/300 [10.2m elapsed, 0s remaining, 0.5 samples/s]
[23]:
from tqdm import tqdm
predictions = []
# Call Qdrant to find the closest data points
for embedding in tqdm(test_embeddings):
prediction = generate_fiftyone_classification(embedding, brain_key="bdd100k_qdrant_example", field="timeofday")
predictions.append(prediction)
test_view.set_values("timeofday", predictions)
100%|ββββββββββ| 300/300 [00:09<00:00, 30.38it/s]
Now we can pull up the FiftyOne App to inspect the timeofday
labels on the test set to inspect how the classifier performed.
[24]:
# Inspect how the annotations look
session = fo.launch_app(test_view)
[26]:
session.show()
[27]:
session.freeze() # screenshot the App
From here, there are multiple ways forward to further refine the preannotations. Two of these paths are:
Bringing in a QA team to use the FiftyOne App to tag mistakes
Using the mistakenness feature of the FiftyOne Brain to automatically detect potential annotation mistakes
Either way, these preannotations can then easily be passed to the annotation tools that FiftyOne integrates with like CVAT, Labelbox, etc. for further refinement.
Other ways to interact with QdrantΒΆ
In these examples, we have shown you how to compute embeddings and load them into Qdrant using compute_similarity. However, you can do many other things with the Qdrant integration as well!
To quickly show you some of this functionality, weβll use th quickstart dataset which contains 200 images.
[2]:
dataset = foz.load_zoo_dataset("quickstart")
print(dataset)
Dataset already downloaded
Loading 'quickstart'
100% |βββββββββββββββββ| 200/200 [1.7s elapsed, 0s remaining, 115.5 samples/s]
Dataset 'quickstart' created
Name: quickstart
Media type: image
Num samples: 200
Persistent: False
Tags: []
Sample fields:
id: fiftyone.core.fields.ObjectIdField
filepath: fiftyone.core.fields.StringField
tags: fiftyone.core.fields.ListField(fiftyone.core.fields.StringField)
metadata: fiftyone.core.fields.EmbeddedDocumentField(fiftyone.core.metadata.ImageMetadata)
ground_truth: fiftyone.core.fields.EmbeddedDocumentField(fiftyone.core.labels.Detections)
uniqueness: fiftyone.core.fields.FloatField
predictions: fiftyone.core.fields.EmbeddedDocumentField(fiftyone.core.labels.Detections)
Note: Each of the following sections can be run independently of each other and in any order.
Use an existing indexΒΆ
Suppose you already have a Qdrant collection storing the embedding vectors you need for the samples or patches in your dataset. You can connect to them directly by passing in the collection_name
parameter to compute_similarity().
[3]:
fob.compute_similarity(
dataset,
model="clip-vit-base32-torch", # zoo model used (if applicable)
embeddings=False, # don't compute embeddings
collection_name="your-collection", # the existing Qdrant collection
brain_key="existing_qdrant_index",
backend="qdrant",
)
[3]:
<fiftyone.brain.internal.core.qdrant.QdrantSimilarityIndex at 0x7fb4aa2d4160>
Add or remove embeddings from an indexΒΆ
Now suppose you have made changes to your dataset that involve removing or adding samples. You would need to then modify your embeddings in Qdrant to also reflect these changes. You can use add_to_index() and remove_from_index() to add or remove embeddings from an existing Qdrant index.
[4]:
import numpy as np
qdrant_index = fob.compute_similarity(
dataset,
model="clip-vit-base32-torch",
brain_key="add_or_remove_qdrant_embeddings",
backend="qdrant",
)
print("Starting Index Size: " + str(qdrant_index.total_index_size)) # 200
view = dataset.take(10)
ids = view.values("id")
# Delete 10 samples from a dataset
dataset.delete_samples(view)
# Delete the corresponding vectors from the index
qdrant_index.remove_from_index(sample_ids=ids)
print("Post Delete Index Size: " + str(qdrant_index.total_index_size)) # 190
# Add 20 samples to a dataset
samples = [fo.Sample(filepath="tmp%d.jpg" % i) for i in range(20)]
sample_ids = dataset.add_samples(samples)
# Add corresponding embeddings to the index
embeddings = np.random.rand(20, 512)
qdrant_index.add_to_index(embeddings, sample_ids)
print("Post Addition Index Size: " + str(qdrant_index.total_index_size)) # 210
Computing embeddings...
100% |βββββββββββββββββ| 200/200 [15.6s elapsed, 0s remaining, 13.6 samples/s]
Starting Index Size: 200
Post Delete Index Size: 190
100% |βββββββββββββββββββ| 20/20 [5.5ms elapsed, 0s remaining, 3.6K samples/s]
Post Addition Index Size: 210
Since we modified this dataset, weβre going to reload it so that it works as expected for the remaining examples.
[5]:
dataset = foz.load_zoo_dataset("quickstart", drop_existing_dataset=True)
Dataset already downloaded
Deleting existing dataset 'quickstart'
Loading 'quickstart'
100% |βββββββββββββββββ| 200/200 [1.8s elapsed, 0s remaining, 108.5 samples/s]
Dataset 'quickstart' created
Retrieve embeddings from an indexΒΆ
Do you have embeddings already calculated on a dataset that you want to retrieve in full? Or perhaps you have a view on your dataset that you want embeddings for? Use the function get_embeddings() to get your embeddings from a Qdrant index by ID.
[6]:
qdrant_index = fob.compute_similarity(
dataset,
model="clip-vit-base32-torch",
brain_key="qdrant_index",
backend="qdrant",
)
# Retrieve embeddings for the entire dataset
ids = dataset.values("id")
embeddings, sample_ids, _ = qdrant_index.get_embeddings(sample_ids=ids)
print(embeddings.shape) # (200, 512)
print(sample_ids.shape) # (200,)
# Retrieve embeddings for a view
ids = dataset.take(10).values("id")
embeddings, sample_ids, _ = qdrant_index.get_embeddings(sample_ids=ids)
print(embeddings.shape) # (10, 512)
print(sample_ids.shape) # (10,)
Computing embeddings...
100% |βββββββββββββββββ| 200/200 [15.6s elapsed, 0s remaining, 12.2 samples/s]
(200, 512)
(200,)
(10, 512)
(10,)
Access the Qdrant client directlyΒΆ
While FiftyOne provides many ways to interact with Qdrant, sometimes you just need to connect directly to get your task done. Thus, you can directly access the Qdrant client instance by using the client
property of a Qdrant index. From there, you can use the Qdrant methods as desired.
[7]:
qdrant_index = fob.compute_similarity(
dataset,
model="clip-vit-base32-torch",
brain_key="access_qdrant_client",
backend="qdrant",
)
qdrant_client = qdrant_index.client
print(qdrant_client)
print(qdrant_client.get_collections())
Computing embeddings...
100% |βββββββββββββββββ| 200/200 [15.5s elapsed, 0s remaining, 13.2 samples/s]
<qdrant_client.qdrant_client.QdrantClient object at 0x7fb4e8f2c550>
collections=[CollectionDescription(name='fiftyone-quickstart'), CollectionDescription(name='fiftyone-quickstart-0czljr'), CollectionDescription(name='fiftyone-quickstart-9x04wr'), CollectionDescription(name='fiftyone-bdd100k-validation-1000-9dll7r'), CollectionDescription(name='fiftyone_docs'), CollectionDescription(name='fiftyone-quickstart-40zten'), CollectionDescription(name='fiftyone-quickstart-183n07'), CollectionDescription(name='fiftyone-mnist-2500'), CollectionDescription(name='fiftyone-bdd100k-validation-1000'), CollectionDescription(name='fiftyone-mnist-2500-ais83e'), CollectionDescription(name='fiftyone-quickstart-jg78it'), CollectionDescription(name='fiftyone-quickstart-c2w0tp'), CollectionDescription(name='fiftyone-quickstart-bsjo0g'), CollectionDescription(name='fiftyone-mnist-2500-sipg85'), CollectionDescription(name='fiftyone-quickstart-tlu0iq'), CollectionDescription(name='fiftyone-quickstart-9jv2wl')]
And more!ΒΆ
To read up on everything you can do with the FiftyOne Qdrant integration, check out the Qdrant integration page in our docs!
SummaryΒΆ
FiftyOne and Qdrant can be used together to efficiently perform a nearest neighbor search on embeddings and act on the results on your image and video datasets. The beauty of this process lies in its flexibility and repeatability. You can easily load additional ground truth labels for new fields into both FiftyOne and Qdrant and repeat this pre-annotation process using the existing embeddings. This can quickly cut down on annotation costs and result in higher-quality datasets, faster.